Forensic Identity to Secure Cannabis Photos: Hytiva's Innovative Approach
Hytiva's Cannabis Photo Identification tool safeguards their cannabis images with unique fingerprints and advanced watermarking, ensuring authenticity and preventing theft.
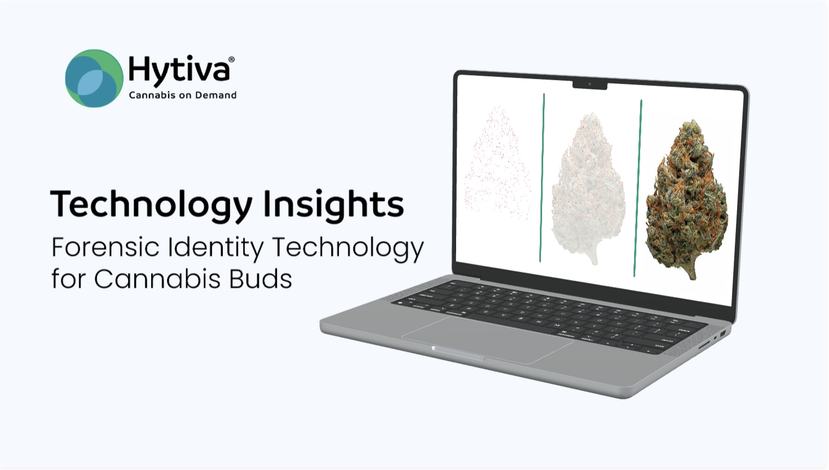
In the world of technology and innovation, it's always thrilling to see new solutions emerge to tackle pressing problems. Hytiva®, a leading name in the cannabis industry, has done something remarkable. They've built the largest documented collection of cannabis flower images, investing significant time and money to purchase each strain. These images are a valuable asset for their clients, who use them on their menus, in store displays, interactive experiences, and more. Recognizing the importance of protecting this asset, Hytiva Technologies, under the leadership of CEO Tom Clarke and CTO Daniel Gozick, devised a groundbreaking solution: Hytiva’s Cannabis Photo Identification tool. This tool creates a unique fingerprint for each cannabis bud, ensuring the images' authenticity and protecting them from theft.
The concept of fingerprinting a bud might sound straightforward, but it actually requires a complex process that leverages multiple advanced image comparison techniques, metrics, and thresholds to accurately measure the similarity between two images. This process is further complicated by various factors, including compression, contrast, brightness, rotation, and semantic identity, which can significantly impact the results.
Image Comparison Methods
-
Mean Absolute Error (MAE)
MAE measures the average absolute difference between corresponding pixels in two images, providing a simple and intuitive measure of similarity. However, it can be sensitive to outliers and may not accurately capture nuanced differences between images. -
Mean Squared Error (MSE)
MSE calculates the average squared difference between corresponding pixels in two images, penalizing large errors more heavily than small ones. This makes it suitable for applications where small errors are tolerable, but large errors are not, such as image compression or denoising. -
Peak Signal-to-Noise Ratio (PSNR)
PSNR measures the ratio of the maximum possible power of a signal to the power of corrupting noise, providing a widely used metric for evaluating image quality. It is particularly useful for comparing images with different compression levels or artifacts, but may be less effective for comparing images with different content or structures. -
Normalized Cross-Correlation (NCC)
NCC measures the similarity between two images by calculating the correlation coefficient between corresponding pixels, providing a robust measure of similarity that is insensitive to intensity differences. It is particularly useful for applications such as image registration, object recognition, or tracking, where images may have different lighting conditions or viewpoints.
Standardization
Now that we have a framework for comparing images, we can begin preparing them for analysis by standardizing their format, which is a crucial step in ensuring accurate and reliable results. This process, known as pre-processing, involves two key steps:
-
Standardize Image Size
We resize all images to a uniform size, which significantly reduces computational complexity and makes subsequent steps more efficient. -
Convert to Suitable Formats
Next, the images are converted to formats that are easier to compare, such as grayscale and RGB channels. This conversion is essential for extracting the features that will later be used for identification.
Identifying Metrics
Having standardized and pre-processed the images, we can now input them into our Photo Identification tool, which produces a comprehensive set of metrics that quantify the similarities between the images. By applying predefined rules and thresholds to these metrics, we can automatically flag images that require further evaluation, capturing key data points about each image, which includes:
-
Image Metadata
Collects metadata associated with the infringing image, such as the URL, timestamp, and image dimensions. -
Image Preview
Provides a preview of the infringing image, allowing you to quickly verify the infringement. -
Infringement Details
Provides a cross reference table about the infringement that include the strain name, creation dates, and other industry details.
Watermarking
To provide an additional layer of protection for Hytiva's Intellectual Property, all images undergo a Just-In-Time generation process that incorporates advanced security measures. This process ensures that images are safeguarded against unauthorized use, regardless of their size. Two key features of this process are:
-
Watermarking
The system automatically embeds a subtle watermark into the generated images. -
Hidden Watermark
Utilizing a combination of Discrete Wavelet Transform (DWT) and Discrete Cosine Transform (DCT), the watermark is embedded in a way that preserves the image's quality and integrity. This approach enables reliable detection by algorithms, while maintaining the image's visual appeal.
The Final Result
By implementing these steps, Hytiva’s Cannabis Photo Identification tool effectively safeguards their cannabis photo assets. This comprehensive approach not only protects the images from unauthorized use but also ensures that clients can trust the authenticity of the images they use. Through innovative technology and strategic processes, Hytiva® demonstrates its commitment to maintaining the integrity and value of its documented cannabis flower collection.
In a world where digital assets are increasingly valuable and vulnerable to theft, Hytiva’s proactive approach is a testament to their dedication to quality and security. Their Cannabis Photo Identification tool is not just a technological innovation but a vital component of their business strategy, ensuring that their investment in documenting cannabis flowers remains protected and beneficial to their clients. It’s a brilliant example of how technology can be harnessed to solve real-world problems, maintaining the trust and integrity that Hytiva’s clients rely on.
The below image demonstrates a visual comparison, showing the differential of two images and how Hytiva’s Cannabis Photo Identification tool identifies differences:
Building More Than An IP Protection Tool
Using a wealth of experience amassed in facial recognition (including creating unique fingerprints for human faces) and building AI systems applied to facial features, among other uses, Daniel and team used their engineering prowess to create this unique solution. While photo protection provided a straightforward business case for creating this technology, Hytiva’s intentions for this technology were already far greater, including AI identification of buds, and much more. Hytiva® is already using its cannabis bud identification technology for these other purposes to serve its clients and continue pushing cannabis technology forward.